Scientific metadata provide the information necessary for investigators separated by time, space, institution or disciplinary norm to establish common ground. | Edwards, 2011
The structured and standardised metadata that a data archive assigns to a dataset, are an important condition for the realisation of FAIR data. In this section, we will show how different scientific disciplines deal with this.
Assigning metadata
When a dataset is ingested in a data archive, checks are made to establish whether the dataset has been described well enough. The key question is: does a (future) user or computer have sufficient information to be able to find the data and understand what the dataset entails? If not, reuse is unthinkable and reproducibility is a mission impossible.
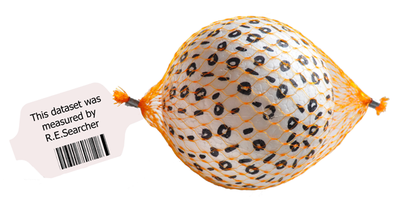
Both the person who archives the data and the data manager of a data archive can assign so-called structured metadata. Which metadata fields are mandatory or desirable differs per data archive and research discipline. Different disciplines use their own metadata schemes and standards for this (RDA, n.d.). The use of such standards is essential to enable the findability, interoperability and reusability of datasets.
Both DANS and 4TU.ResearchData use the Dublin Core Metadata Initiative as metadata standard (DCMI, n.d.). Dublin Core is easy to use and is used worldwide. DataCite (n.d.), the organisation that provides Digital Object Identifiers (DOIs), has drawn up its own metadata standard for datasets with a DOI. This standard - the DataCite Metadata Schema (2019) - is richer than Dublin Core. For example, it offers more possibilities to describe the dataset precisely. Because this standard is becoming increasingly popular, data archives such as DANS and 4TU.ResearchData make it possible for metadata to be 'harvested' in this metadata format by metadata aggregators such as DataCite, which in turn make it possible to search in the harvested metadata and find the corresponding datasets (Also see the section 'Searching for data').
What differs per metadata standard are the agreements about how information is encoded and should be understood. In one metadata standard, for example, the date of publication is shown as 'datePublished' and in another as 'date' or 'PublicationYear'. Or in one metadata standard the geographical coverage is coded as 'SpatialCoverage' and in another as 'GeoLocation'. To ensure that data in a discipline can talk to each other, they must be described using the same metadata standard.
Types of metadata
In the table below, the role of different important types of metadata - which can then be described with different types of metadata standards - is explained.
Metadata are often called 'data about data', or information about information. Metadata exist to describe the content (descriptive metadata) and metadata exist to indicate the context (date of creation, instruments, etc.). Without contextual metadata, some data would appear to be nothing more than a random arrangement of numbers, pictures or words. And without descriptive metadata it is impossible to find relevant data in a data archive.
The most common types of metadata are:
Type of metadata | Goal | Example |
---|---|---|
Descriptive metadata | These are the minimum metadata needed to find a digital object. If contextual metadata are also present, a user gets more insight into how to use the data him- or herself. | Author, title, abstract, date. Contextual metadata are, for example, location, time, methods of data collection (tools). |
Structural metadata | These record the relationship between individual objects that together form a unit. | Links to related digital objects, (e.g. the article written on the basis of the linked research data). |
Technical metadata | Information on the technical aspects of the data. | E.g. data format, hardware/software used, calibration, version, authentication, encryption, metadata standard. |
Administrative metadata | Metadata that focus on usage (rights) and management of digital objects. | E.g. license, possible reasons for an embargo, waivers, search logs, user tracking. |
FAIR metadata is the first major step towards becoming maximally FAIR. When the data elements themselves can also be made FAIR and made open for reuse by anyone, we have reached the highest degree of FAIRness. When all of these are linked with other FAIR data, we will have achieved the Internet of (FAIR) Data. Once an increasing number of applications and services can link and process FAIR data we will finally achieve the Internet of FAIR Data and Services. | Mons et al., 2017
Enriching data
In order to make data usable for other researchers who have not yet worked with the data, it is often not enough to assign standardised metadata. In addition to metadata, all the necessary information required to guarantee usability is also stored in a data archive. Think, for example, of data documentation such as manuals for using software, code books with the abbreviations, variables and codes that occur in data, but also of the software and code itself if it is necessary to perform data analyses. In addition, it is often necessary to add an index of the dataset with a substantive description of the folders and possibly also of the data files themselves (if they do not speak for themselves).
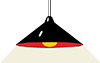
In the spotlight
About metadata schemes and metadata standards
A metadata scheme is a set of individual metadata elements that you can use to describe data. Most schemes are developed and endorsed by certain communities. In a metadata scheme, each metadata element is given a name and meaning. An example of a scheme developed by the community is the Data Documentation Initiative (DDI, n.d.), an international standard for describing data from social science, behavioural science and economic research.
When a standardisation body such as the ISO (n.d.) approves a metadata scheme, it is called a metadata standard. An example of a metadata standard is the Dublin Core Metadata Element Set (DCMI, n.d.b.) also known as ISO 15836-1:2017 (ISO, 2017a) and ISO/DIS 15836-2 (ISO, 2017b).
There are many different metadata schemes and standards, depending on the research community, the purpose, the function and the domain. The Digital Curation Centre provides a good overview of the schemes and standards used within a number of disciplines (DCC, n.d.). RDA also maintains an overview (RDA, n.d.).
Mandatory metadata fields at DANS and 4TU.ResearchData
DANS and 4TU.ResearchData require the following metadata:
DANS | 4TU.ResearchData | Meaning |
---|---|---|
Creator | Creator | The main researchers involved in producing the data |
Title | Title | Name or title of dataset |
Date created | Date created | |
Description | Description | |
Audience | Audience for whom the dataset is interesting, described in terms of areas of research | |
Publication year | ||
Rights holder | The person or organisation that holds the copyright or intellectual property rights | |
Access Rights | A basic choice between Open Access or Restricted Access and a mandatory choice for the type of licence if Open Access is chosen (CC0-1.0; CC-BY-4.0 etc.) |
These are only the mandatory metadata fields. The more metadata fields that are filled in, the better findable and usable the dataset will be.
Making a data package
To publish research data in general data archives such as Figshare (n.d) or Zenodo (n.d.), they are often uploaded in a so-called data package. Such a self-descriptive data package contains the research data itself plus all the information needed to understand and use the data. Finally, there must be a README file in the package and in each folder in which all the files and their mutual relationship are described.
For an example of a data package, take a look at:
- Hardisty, A.R, Belbin, Lee, Hobern, Donald, McGeoch, Melodie A, Pirzl, Rebecca, Williams, Kristen J, & Kissling, W Daniel. (2018). Data package supporting an Invasive Species Distribution (IVSD) workflow for prototype Essential Biodiversity Variable (EBV) data product [Data set]. Zenodo. https://doi.org/10.5281/zenodo.2275703
- Neylon, Cameron. (2017). Dataset for IDRC Project: Exploring the opportunities and challenges of implementing open research strategies within development institutions. International Development Research Center. [Data set]. Zenodo. https://doi.org/10.5281/zenodo.844394
The second example uses, among other things, DataCrate (Sefton, 2019), a specification for creating a data package with human- and machine-readable metadata. Another tool to create FAIR data packages is, for example, Frictionless Data (n.d.), described in a blog (Open Knowledge Foundation, 2018).

Sources
Click to open/close
Angevaare. I (2011). 'Linked Data' - wat is dat nu eigenlijk precies? [blog]. http://digitaalduurzaam.blogspot.com/2011/01/linked-data-wat-is-dat-nu-eigenlijk.html
Crossref (n.d.). Funder Registry. https://www.crossref.org/services/funder-registry/
Cruz, M. J., Kurapati, S., & der Velden, Y. T. (2018, July 6). Software Reproducibility: How to put it into practice?. https://doi.org/10.31219/osf.io/z48cm
DataCite (n.d.). DataCite Search. https://search.datacite.org/
DataCite (2019, Augustus 16th). Datacite Metadata Schema. Metadata Schema 4.4. https://schema.datacite.org/
DCC (n.d.). Disciplinary Metadata. http://www.dcc.ac.uk/resources/metadata-standards
DDI (n.d.). Data Documentation Initiative. Retrieved from http://www.ddialliance.org/
DCMI (n.d.a.). Dublin Core Metadata Initiative. http://dublincore.org/
DCMI (n.d.b.) DCMI Metadata Terms. https://www.dublincore.org/specifications/dublin-core/dcmi-terms/
Edwards, P. (2011). Science Friction: Data, Metadata, Collaboration. Social Studies of Science, 41(5), 667-690. doi:10.1177/0306312711413314
Figshare (n.d.). https://figshare.com/
Frictionless data (n.d.). Data Packages. http://frictionlessdata.io/data-packages/
Hardisty, A.R, Belbin, Lee, Hobern, Donald, McGeoch, Melodie A, Pirzl, Rebecca, Williams, Kristen J, & Kissling, W Daniel. (2018). Data package supporting an Invasive Species Distribution (IVSD) workflow for prototype Essential Biodiversity Variable (EBV) data product [Data set]. Zenodo. https://doi.org/10.5281/zenodo.2275703
ISO (n.d.). https://www.iso.org/home.html
ISO (2017a). INFORMATION AND DOCUMENTATION -- THE DUBLIN CORE METADATA ELEMENT SET -- PART 1: CORE ELEMENTS. https://www.iso.org/standard/71339.html
ISO (2017b). INFORMATION AND DOCUMENTATION -- THE DUBLIN CORE METADATA ELEMENT SET -- PART 2: DCMI PROPERTIES AND CLASSES.https://www.iso.org/standard/71341.html
Mons, B., Neylon, C., Velterop, J., Dumontierf, M.,et al. (2017). Wilkinson Cloudy, increasingly FAIR; revisiting the FAIR Data guiding principles for the European Open Science Cloud. Information Services & Use, vol. 37, no. 1, pp. 49-56. https://doi.org/10.3233/ISU-170824
Neylon, Cameron. (2017). Dataset for IDRC Project: Exploring the opportunities and challenges of implementing open research strategies within development institutions. International Development Research Center. [Data set]. Zenodo. https://doi.org/10.5281/zenodo.844394
Open Knowledge Foundation (2018, August 14). Frictionless Data and FAIR Research Principles. [blog]. https://blog.okfn.org/2018/08/14/frictionless-data-and-fair-research-principles/
RDA (n.d.). Metadata Directory. http://rd-alliance.github.io/metadata-directory/standards/
Sefton P., Lynch M. (2019). Packaging Research data with DataCrate - a cry for help! https://doi.org/10.6084/m9.figshare.8066936.v1
W3C (n.d.). RDF. https://www.w3.org/RDF/